The Orlando Magic, Dan Altman, and why we should walk before we run
Preaching to the choir over here...
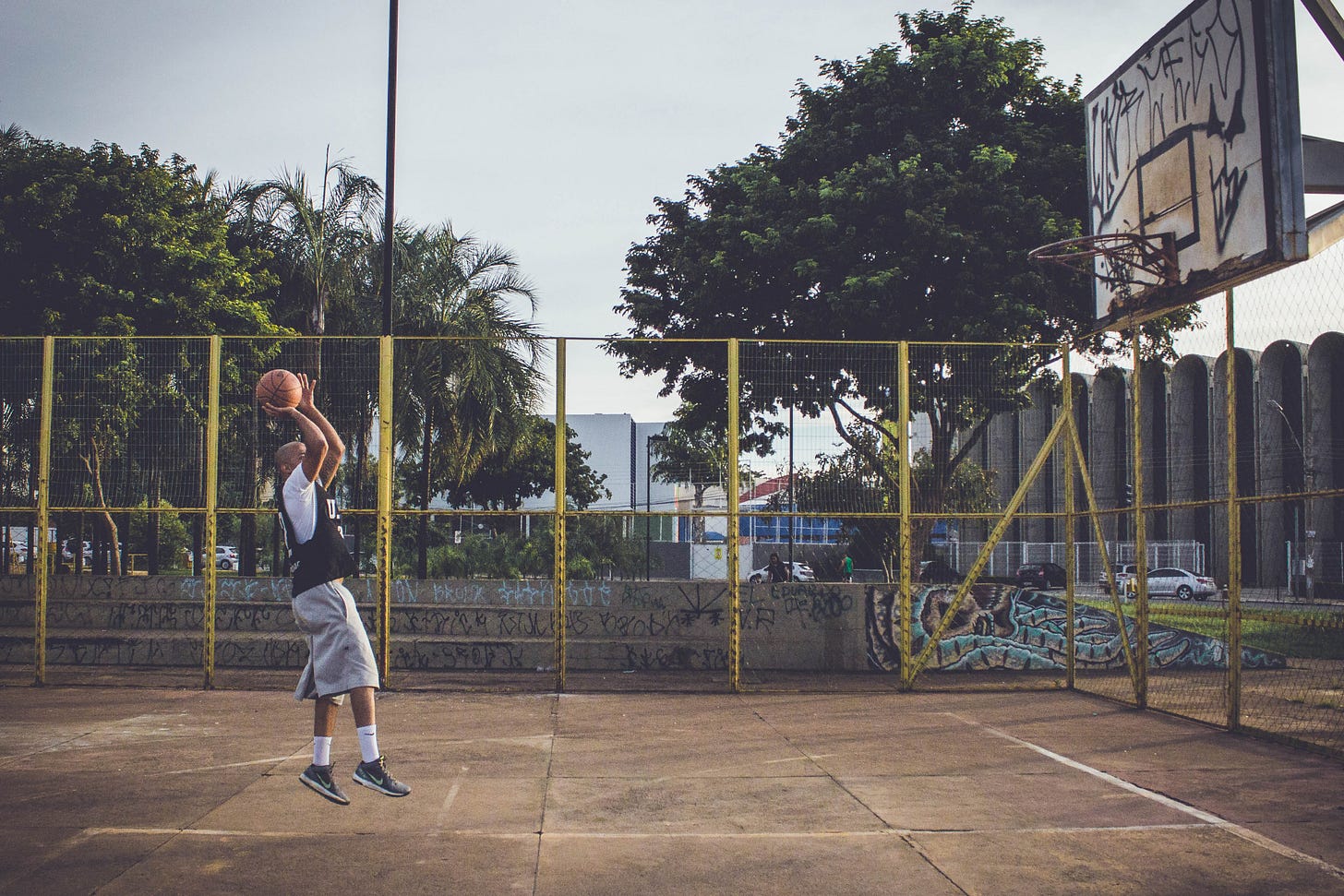
Photo by Justiniano Adriano from Pexels
A few days ago, a headline caught my eye in a daily sports science newsletter I receive: “Orlando Magic Are Pioneers In Introducing Artificial Intelligence To NBA Scouting.” The article details the Magic’s partnership with STATS in applying AI to broadcast feed-based tracking data in using OpenPose, which, according to STATS, “‘unlocks new layers of body-pose information, providing a deeper quality of player tracking data like body position, shot form, torque and other aspects of the game.’”
Me being myself, I was immediately skeptical; when most teams struggle to even put into place a set of basic best practices when it comes to recruitment, are we really at the stage where we need to leap forward to applying AI to scour tracking data?
However my beat isn’t basketball, and the NBA tend to be leaders in this area. But when I read paragraphs like this from the article, more than a few questions come to mind.
Although technology and its place in player evaluation can be a polarizing issue, OpenPose could be a game changer. If the results are proved accurate, a longstanding subjective criterion such as shooting mechanics might finally be flipped objective. That’s intriguing for a front office.
But as with many other industries threatened with automation, that could be bad news for the small community of scouts and others in basketball operations. As front offices have continued to grow around the league, owners will undoubtedly be tasked with choosing whether to keep large staffs or go the way of artificial intelligence.
So the questions I’m asking myself are: do we know whether OpenPose has already identified markers related to improved shooting mechanics (or defensive mechanics for that matter)? Do we know how predictive these measures are, or repeatable, or consistent in certain players, which would make it valuable in recruitment? Or are these markers translatable into coaching techniques? Can you make a good player great through actionable insights based on the technology?
And if we, the public, don’t know this yet, does the Magic?
If they don’t, I suppose the Magic could justify the investment with the understanding, “we’ll see, hopefully before the next autoSTATS client does.” But if I worked for the team, I would be far less interested in the potential of this technology, but whether those who have developed it can demonstrate the value of any insights it has already produced, whether to sports science or to recruitment, and how we can realistically integrate that into our team process.
And then today, I read this tweet, a quote from very smart person Luke Bornn:

This is in line with what I’m getting at here. And before you start wondering whether this is still, in fact, a soccer analytics newsletter, Bornn’s thinking on this matter also echoes the words of Dan Altman, formerly a consultant at Swansea City and creator of smartscout, who spoke this past week with Paul Riley for the latter’s blog on his experiences working with the Swans:
Do you think the turnover in managers at the club contributed to ideas not being taken up or are we talking about getting decisions implemented at executive level?
I worked for several years as a strategic consultant before getting into football, and I have some ideas about how organisations ought to run and how decision making processes ought to look. I feel very strongly that even if you didn’t have an analyst at all, if you had a good decision making process, you’d probably make better decisions than a club with a great analyst and no process. That was one of the things I thought could improve at Swansea.
What kind of structure should be in place?
Any club should have regular meetings at middle management level for tactics and recruitment and regular, less frequent meetings about those things at a higher level. I think that decisions in that context can be consensus-based, where you are able to collect input from all the relevant parties and keep everybody informed about what’s going on.
Italics mine. As Paul himself acknowledges, this all sounds like common sense! Yet the fact many clubs still put the cart before the horse in hiring analysts before understanding how to implement their work into the overall team process speaks to something I touched on last week, and a common trope in the sports analytics business.
This is the problem of clubs not being able to understand the value-add of data and analytics before they invest in them, i.e. how are non-data scientists in a position to separate analytics snake oil from the good stuff?
I think this is a real issue, but I also believe that a club with intelligent decision-making processes in place will ask the right questions of analysts as a matter of course.
So, for example, a team with a decent player recruitment process will probably want some concrete information from potential analytics hires, such as (and this is off the top of my head):
How precisely will your model improve our overall success rate in recruitment?
Does your model provide KPIs across all positions and for defensive and attacking ability?
How does your model perform among players with a transfer fee or wages within these limits?
How would your work assist us in deciding between two similar players in a particular position, and on what basis?
How successful is your model in separating team effects from individual impact?
How agile is your model, and how complex/expensive are the data which drive it?
Do you have access to enough event or tracking data for players in our realistic target markets?
For a team that is serious about maximizing ROI in player recruitment, these will be very basic questions indeed. And because analysts will spend a significant amount of time on building databases and cleaning data anyway, it shouldn’t be up to them to figure out how their work should fit into the team process—this is the job of the technical director or director of football or chief executive or whatever.
All this again underlines why I think our collective obsession with analytics ignores the problem that even now, in 2019, some clubs insist on running before they walk. And if that’s the case, no amount of sophisticated technology is going to help improve the situation. In other words, let’s talk far less about data science and more on what a good process looks like, which can (and in some cases should) be analytics-agnostic.